Music and text are similar in the way that both of them can be regraded as information carrier and emotion deliverer. People get daily information from reading newspaper, magazines, blogs etc., and they can also write diary or personal journal to reflect on daily life, let out pent up emotions, record ideas and experience. Same power could come from music! Composers express their feelings through music with different combinations of notes, diverse tempo, and dynamics levels, as another version of language. All these similarities drive people to ask questions like:
- Could music deliver information tantamount to text?
- Can we efficiently use text mining approach in music field?
- Why music from diverse culture can bring people so many different feelings?
- What’s the similarity between music from different culture, or composers, or genres?
- To what extend do people grasp the meaning behind each piece of music expressed by the composer?
Take the tragedy Titanic as an example, we learn the tragedy from the newspaper and feel anguished, but we can also get the mourning from the song My Heart Will Go On. The melody contains a lot of minor keys (e.g. $D\flat$, $F\sharp$, $A\flat$), which are more likely to trigger the dissonance via two closely spaced notes hitting the ear simultaneously and thus to make people feel sad.
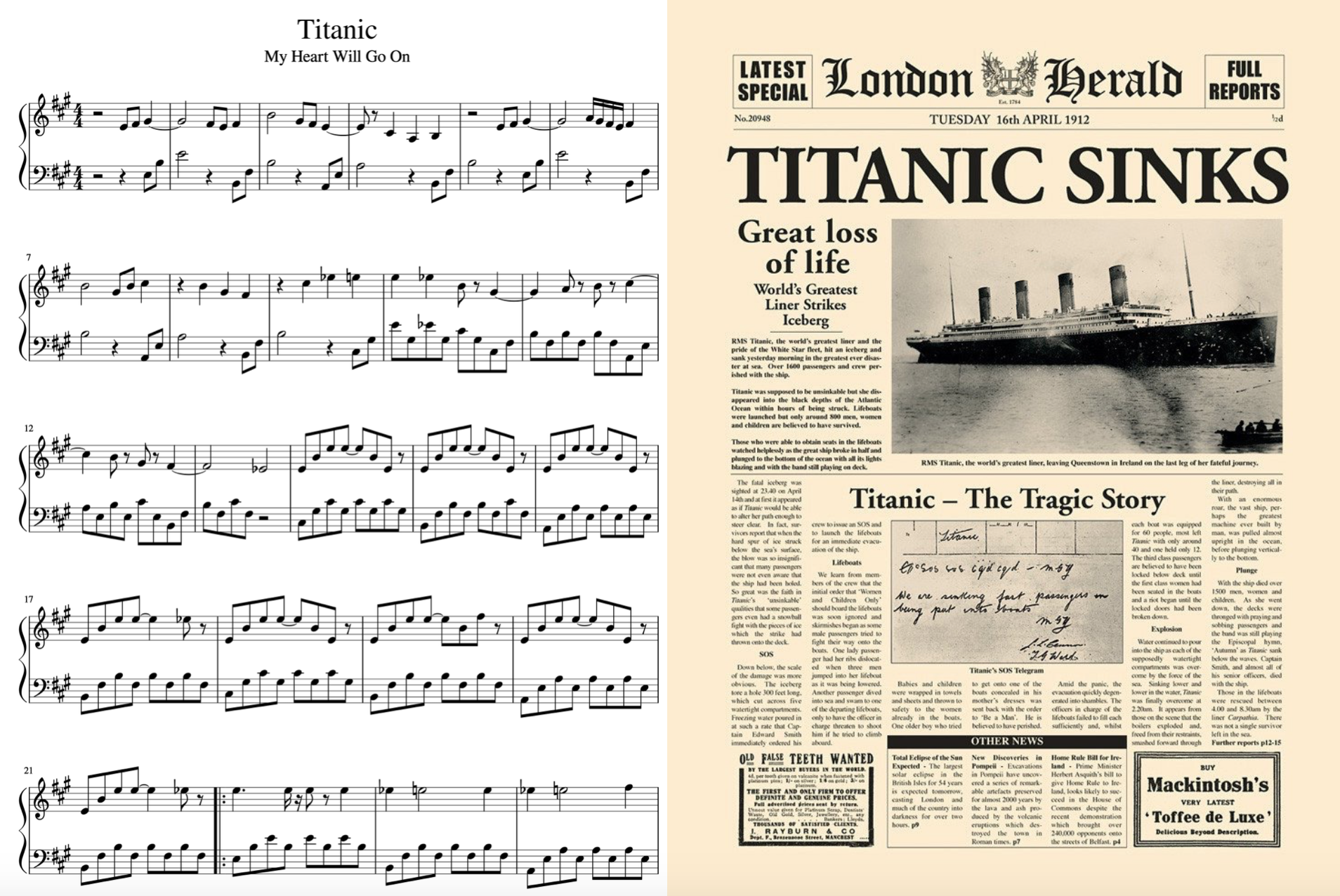
In this project we employ latent Dirichlet allocation model into the music concept. Assume an album, as a collection of songs, are the mixture of different topics (melodies). These topics are the distributions over a series of notes (left part of the figure). In each song, notes in every measure are chosen based on the topic assignments (colorful tokens), while the topic assignments are drawn from the document-topic distribution.
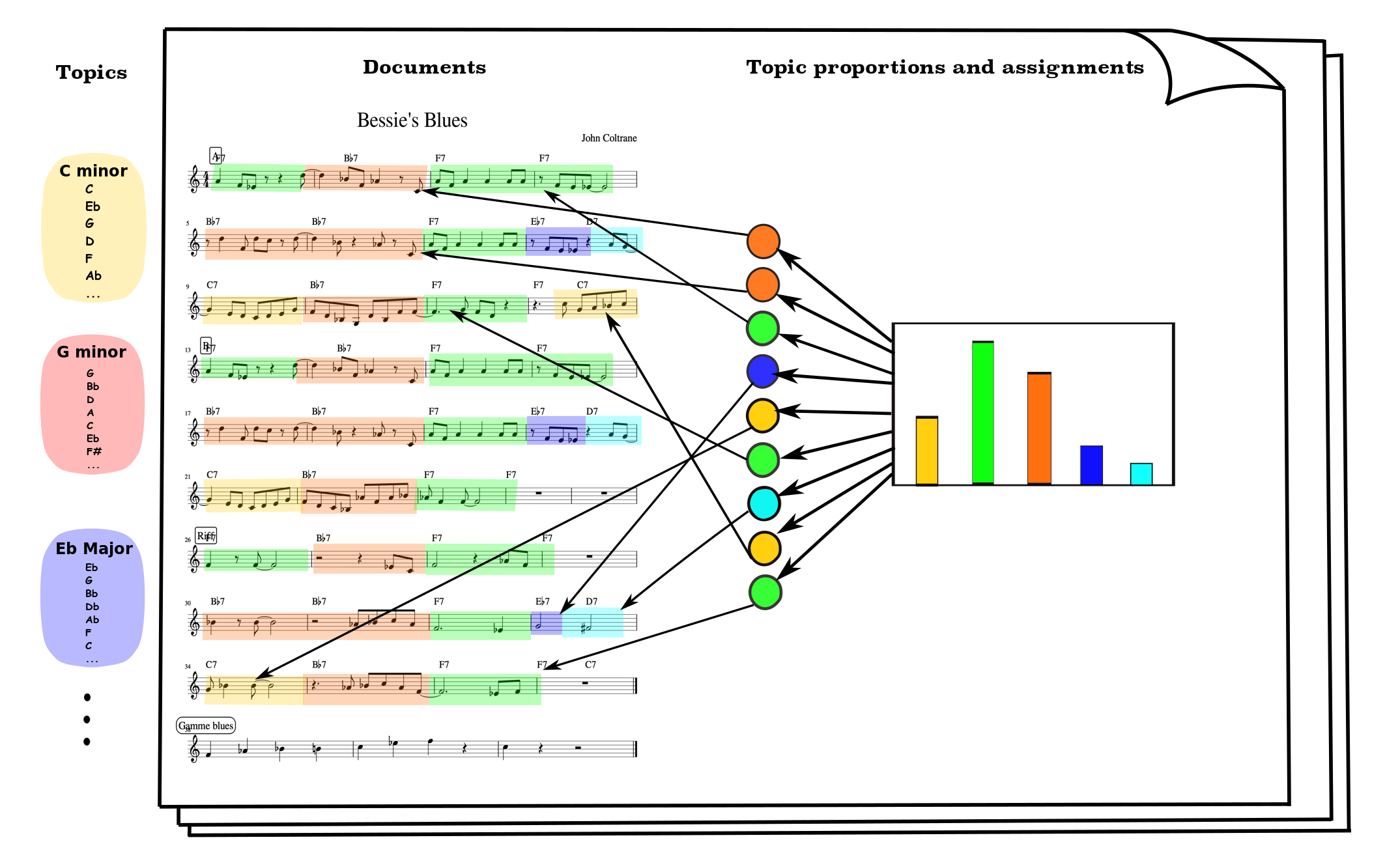
More details can be found in my paper and thesis.
All rights reserved © Copyright 2018, Qiuyi Wu.
Recommended Citation:
[1] Wu, Qiuyi, and Ernest Fokoue. “Naive Dictionary On Musical Corpora: From Knowledge Representation To Pattern Recognition.” arXiv preprint arXiv:1811.12802 (2018).
[2] Wu, Qiuyi, “Statistical Aspects of Music Mining: Naive Dictionary Representation” (2018). Thesis. Rochester Institute of Technology. Accessed from https://scholarworks.rit.edu/theses/9932